Blood Cancer: Could Artificial Intelligence Be the Breakthrough?
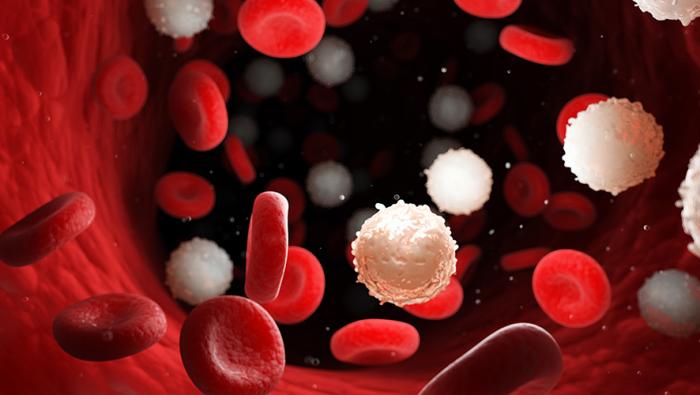
In Dr. Binyamin Knisbacher's laboratory at the Dangoor Center for Personalized Medicine, there are no microscopes. Nor are there test tubes. Instead, there are computers and access to cloud computing, where hundreds of terabytes of genetic data are stored. Using machine learning tools and advanced analysis of genetic sequences, Dr. Knisbacher is attempting to decipher the secret code of blood cancer, developing computational methods for early diagnosis and precise characterization to ultimately enable personalized treatments.
Could the future of blood cancer treatment development lie in the cloud? We spoke with Dr. Knisbacher to learn exactly what’s happening in his unique laboratory at the Faculty of Life Sciences at Bar-Ilan University, and whether the breakthrough might come from machine learning.
"A research direction that is non-standard in genomic research"
To understand how computational research advances cancer treatment, we need to go back to the very beginning – the description of cancer at the cellular level.
Dr. Knisbacher, how does cancer manifest at the cellular level in the body?
"Cancer is a disease of the genome. A single cell accumulates a random mutation, begins to proliferate uncontrollably, and eventually spreads from the original tissue to other tissues, disrupting every tissue it reaches. My field, called computational genomics, seeks to understand the specific disruption cancer causes to each patient's cellular genomic mechanisms in order to identify its weak points and tailor suitable treatments."
This falls under the field of immunotherapy, doesn't it?
"Part of it is actually an effort to advance immunotherapy, which is cancer treatment using the immune system. Today, there are various treatments that can activate the immune system against tumors, and one of our goals is to understand which patients might benefit from these existing methods. Additionally, we aim to expand this treatment approach to new patients by identifying new targets on cancer cells for attack."
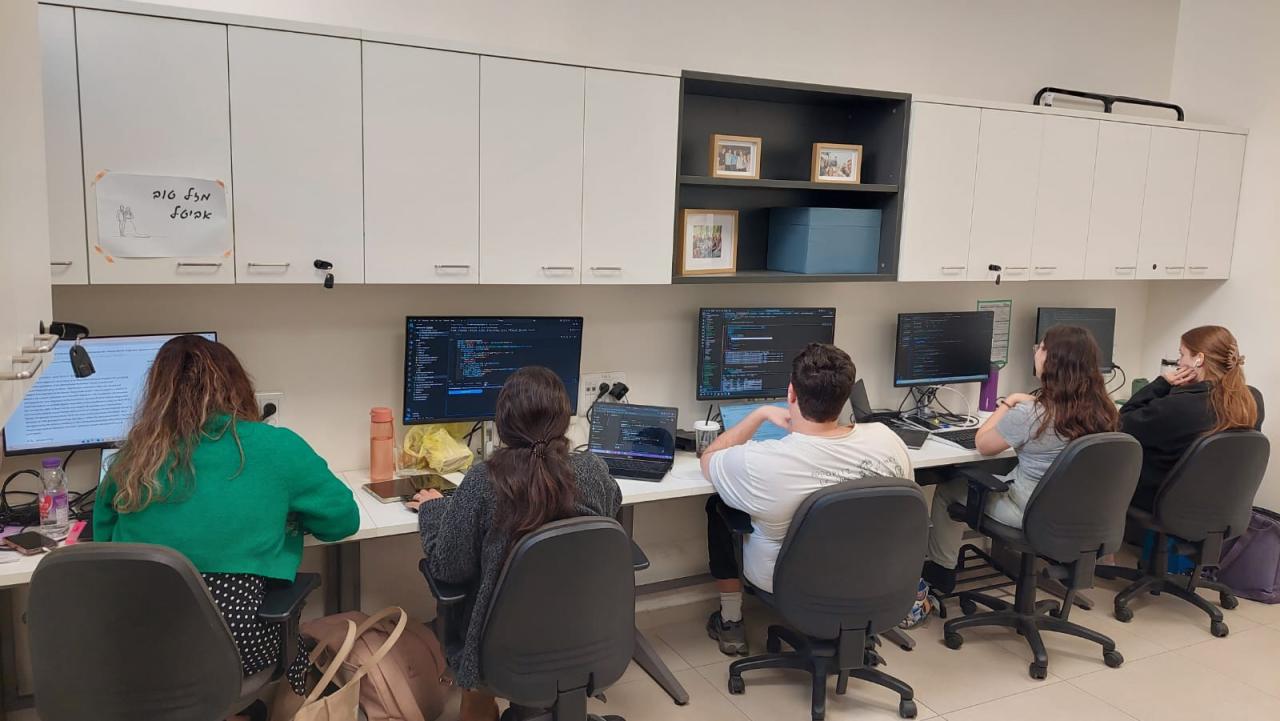
How does this connect to computational genomics?
"When a foreign entity invades the body, it carries a molecule called an antigen, which triggers the body's immune response. Naturally, the cells in our body don't have antigens that would provoke the immune system. However, in cancer, there are cases where a mutation in the DNA creates a new antigen, a neoantigen, which should trigger an immune response, but the cancer still manages to block the immune system and evade it. An innovative immunotherapy called checkpoint inhibitors removes this blockage, allowing the immune system to attack the cancer. To determine which patients could benefit from this treatment, computational genomics is essential. It helps identify neoantigens created by DNA mutations and the methods cancer uses to evade the immune system."
But I noticed you're also identifying disruptions in RNA?
"Correct. Our research direction isn't entirely standard in the field of genomic research. Instead of focusing solely on DNA mutations, as most other studies do, we work on a variety of additional molecular features, particularly RNA. Today, research typically focuses on DNA mutations because they explain most cancers. However, there are types of cancer, such as the blood cancers we focus on, where there are very few DNA mutations. In fact, in certain blood cancers, more than 5% of patients show no DNA mutations associated with the disease. Even when DNA mutations are present, they usually don't tell the whole story."
How does focusing on RNA influence your research?
"RNA is an intermediate molecule between the genetic information stored in DNA and the cell's activity. While DNA is fairly uniform across different cells in the body, RNA varies significantly between tissues. When cancer develops, profound changes occur in RNA, offering us a much more comprehensive view of the genomic disruptions in cancer cells. It complements the DNA picture for us and is ideal for research in terms of cost-effectiveness, as RNA data is relatively inexpensive to process. Some studies even show that predicting cancer's response to treatment is more effective when focusing on RNA rather than DNA, as RNA is closer to the cell's activity."
Does that mean you're not focusing solely on RNA?
"That's correct. Alongside DNA and RNA, we also work with additional layers of information, integrating genetic data with epigenetic information – meaning the factors that regulate gene activity. This gives us a much more complete picture of the cancerous disruptions occurring at the cellular level. Integrating this information allows us to identify RNA and epigenetic disruptions that, from the cell's perspective, lead to the same outcome as a DNA disruption. This is particularly significant because, in patients whose DNA shows no mutations, disruptions in RNA or epigenetics can result in the same cellular effects as DNA mutations."
And does this make it possible to find more effective treatments?
"Yes, it's called targeted biological therapy. This refers to targeted drugs that can bind to a specific protein active in cancer but less so, if at all, in normal cells. For example, there are mutations that cause certain enzymes in the cell to become highly active, which cancer cells depend on, while healthy cells rely on these enzymes much less. As a result, drugs have been developed to inhibit these enzymes. Based on the disruptions observed in RNA expression, we aim to identify which patients could benefit from existing treatments of this kind or propose innovative new targeted biological therapies."
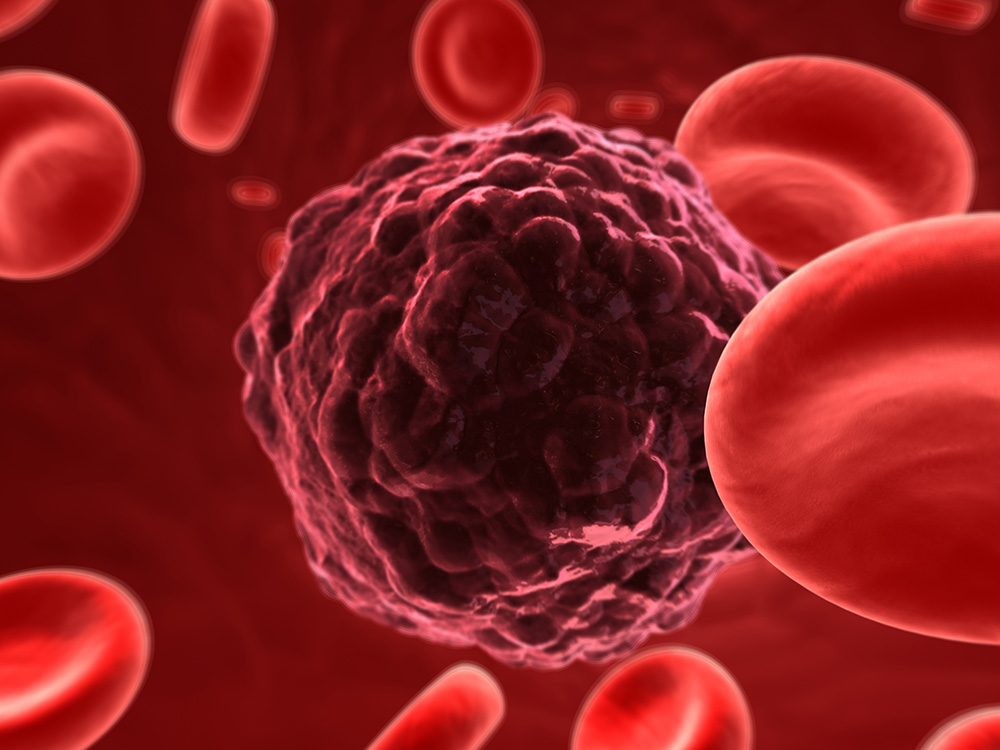
Data spanning hundreds of terabytes
Which type of blood cancer are you working on?
"There are different types of blood cancers. We are studying Chronic Lymphocytic Leukemia, abbreviated as CLL, which affects white blood cells called B cells. Normally, these cells fight infections, but in a cancerous state, they proliferate uncontrollably. However, this is a relatively slow-growing and less aggressive cancer. Clinically, it is often said that one-third of patients will not require treatment at all, one-third will need immediate treatment, and one-third will require monitoring in case treatment becomes necessary later on."
Why did you choose to work on a less aggressive cancer?
"For us, the fact that it's a relatively slow-growing cancer is an advantage because it allows us to follow patients over many years. Additionally, since it's a blood cancer, a simple blood sample is sufficient, without the need for invasive surgery like in other cancers. Alongside the samples from patients, we always strive to also rely on blood samples from healthy individuals to provide a reference point that helps us determine which recurring features in CLL patients are indeed related to the disease."
We interviewed Prof. Sol Efroni, who spoke about the difficulty of obtaining blood samples for research. Do you face a similar challenge?
"Obtaining samples is generally challenging, but even though blood cancers are relatively rare – certainly compared to breast or lung cancer – CLL is the most common blood cancer. As a result, blood samples are routinely collected from all patients over the years. We also work directly with medical centers that hold these samples, so in this case, obtaining blood samples is relatively easy."
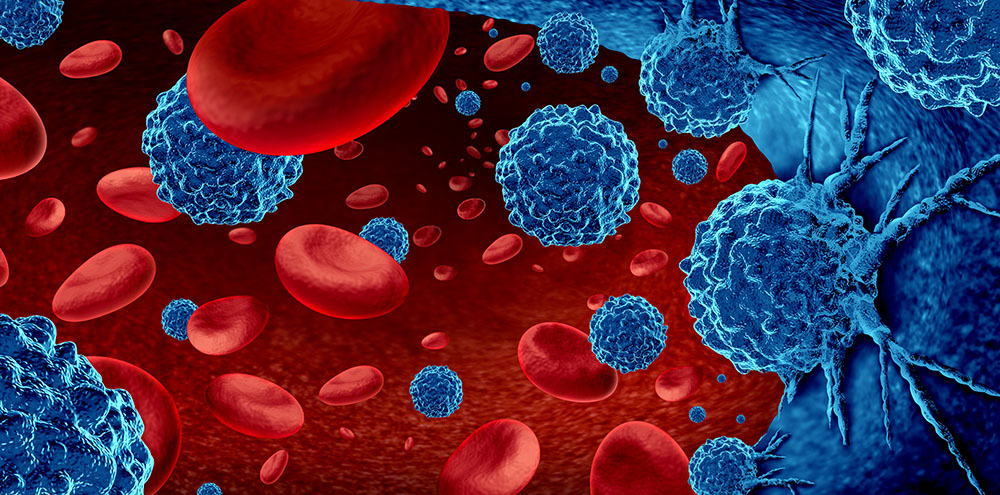
How many samples are we talking about?
"Our research is based on big data, leveraging the ability to study a large number of patients. During my postdoctoral work, we created a large database of over 1,000 patients, which allowed us to identify mutations that had previously been missed. Whereas earlier studies identified mutations recurring in 5% of patients, the larger number of samples now enables us to detect mutations occurring in just 1% of patients."
These are volumes of data that necessitate the use of artificial intelligence.
"Absolutely. Our raw material consists of DNA and RNA sequences, many of them short sequences generated by sequencing machines, which we compare to a reference genome to interpret the situation inside the cell. We analyze the entire genome without predefining which protein to focus on, interpreting the data using our computational capabilities. Using statistical methods, we can identify anomalies between groups of patients and between them and healthy individuals. Through machine learning, we've identified, for instance, groups with drastically different survival rates, and we determine the characteristics of these patient groups so that we can provide doctors with tools to diagnose patients and tailor treatments to each individual."
What are the practical implications of your research – when do you think we might see treatments based on the methods you've developed?
"Our research provides targets for biological and immunotherapy treatments. For example, we identify many immune system characteristics, classify patients into groups, and try to determine which patients are likely to respond well to immunotherapy so that doctors can tailor treatments to each group. Currently, immunotherapy doesn't work well for blood cancer like CLL, and one of the questions we're exploring is whether there is a subgroup for whom this treatment could be effective. Another area is the development of personalized cancer vaccines."
Wait, are you saying there could be a vaccine against cancer?
"It's not a preventive vaccine, like the COVID-19 vaccine, but rather therapeutic vaccines that train the immune system to respond effectively to the disease after it already exists in the body. In this method, proteins resembling the neoantigens found on cancer cells are injected into the body along with a substance that stimulates an immune response, allowing the immune system to learn how to combat the cancer itself. Our role is to identify the cancer-specific neoantigens suitable for this process."
Is there potential for curing other diseases?
"Yes, for example, rare diseases. In fact, one of the students in our lab is collaborating with Sheba Medical Center on a project involving rare diseases in the Clinical Genomics Unit. There, too, we focus on diagnosing genetic diseases through abnormalities observed in RNA."
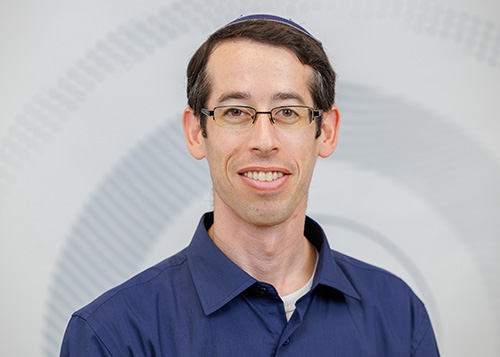
The Future: A sequenced personal genome for everyone
Dr. Knisbacher, 39, completed all three of his degrees, including his doctorate, at Bar-Ilan University, where he essentially grew up within the Computational Biology program where he now teaches. He is married to Rachel and the father of six. He completed his postdoctoral fellowship at the Broad Institute in Boston, a leading global center for genomics research, particularly cancer genomics, where many researchers from Harvard and MIT collaborate.
"In my doctorate, which focused on computational genomics under the guidance of Prof. Erez Levanon, I began exploring cancer research, but the main focus was on more general processes that accelerate genetic changes overall. It was only during my postdoctoral fellowship that I started specializing in computational cancer genomics. During my three years in the U.S., there were significant advancements in the field, particularly through machine learning, and I acquired tools that allowed me to introduce innovative methods into the research we conduct at Bar-Ilan University."
Which academic groups do you collaborate with on your research?
"I'm in contact with a group of doctors and researchers in Israel working on CLL leukemia, and we meet regularly to exchange information. Additionally, I continue to maintain connections with researchers from Harvard and others who have established labs in places like Cornell University, Yale, and even in Austria. There are also many collaborations with hospitals, and fortunately, Bar-Ilan University's physical location makes these partnerships convenient."
Bar-Ilan University is essentially your academic home. What makes it special?
"For me, it's a workplace where you truly feel at home. There's a positive and supportive environment among the researchers and the administration, and there's a sense that everyone shares the common mission of advancing research and teaching, doing whatever they can to help one another. The Faculty of Life Sciences has a wide variety of researchers, offering great potential for fruitful encounters and collaborations."
To wrap up, give us a glimpse of the next ten years—what will personalized medicine look like?
"I think we're heading toward a future where everyone will have a sequenced personal genome. I’d even bet this will happen within twenty years or sooner, as the cost has become so affordable. Today, you can sequence a person’s entire genome for $200, with the main expense being its analysis. Once such mappings become routine, we’ll be able to diagnose and classify patients with precision and tailor their treatments much more effectively."
Visit Dr. Knisbacher's laboratory website: https://www.knisbacherlab.org/
Last Updated Date : 20/01/2025