How Do You Diagnose Cancer From a Table with 2 Million Rows?
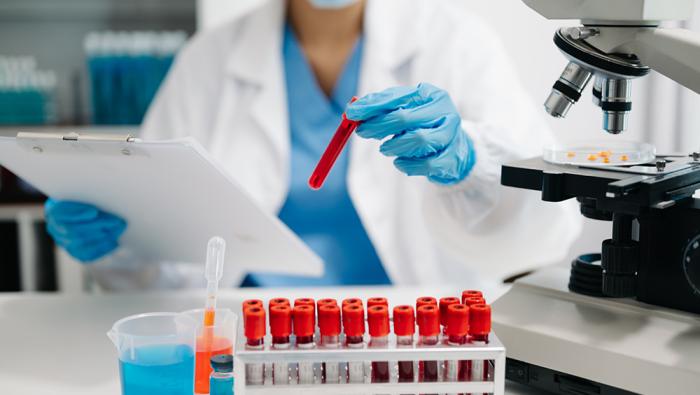
Prof. Sol Efroni from the Dangoor Center for Personalized Medicine and the Faculty of Life Sciences at Bar-Ilan University explains how machine learning tools, such as those underlying Chat-GPT, enable his lab to analyze blood samples and lead to early detection of ovarian cancer and breast cancer
How are machine learning tools used to detect cancer from a blood sample? Prof. Sol Efroni’s Computational Biology Lab at the Dangoor Center for Personalized Medicine and the Faculty of Life Sciences at Bar-Ilan University leverages the tremendous advancements in artificial intelligence to teach the computer to independently analyze blood samples and successfully determine if there is evidence of the early development of cancerous cells.
To understand exactly how artificial intelligence manages to do this, one must first recognize that cancer triggers changes that are identified by the immune system, including by T cells. One of the functions of T cells in our blood is to gather intelligence about the body for the immune system. “It is not yet fully known how they do this, but they receive signals about unusual events through their receptor (T-cell receptor). In addition, once they detect a cancerous cell, they kill it.”
So, can we think of T cells as the body’s Unit 8200?
“You could say that. Some of their functions are like Unit 8200, and others are more like the executive arm. We eavesdrop on the signaling system that activates the T cells by taking a blood sample and examining the T cells within it. In one milliliter of blood, there are 2 million T cells, and they hold a vast amount of information.”
So, do T cells know about the cancer?
“At least in some cases, yes. There’s a game of evasion between cancer and the immune system, and that game is the essence of cancer. According to some theories, cancer is constantly forming, and the immune system is constantly eliminating it. The disease arises when cancer regulation breaks down, or when the immune system fails to kill the cancerous cells. Often, the immune system reaches cancerous or pre-cancerous tumors – meaning, it detects the tumor, but it doesn’t succeed in eliminating it.”
How does it happen that the immune system knows about the tumor but can’t eliminate it?
“The cancerous tumor also takes advantage of the immune system, which supplies it with things it needs – like blood supply and more. There’s an entire field of research called Tumor Microenvironment (TME), which, among other things, studies how cancer uses the immune system. There are cases where the immune system knows the tumor is there, but the tumor signals to it: ’you have no business here, you’ve got the wrong direction.’ Recently, a subfield of immunotherapy has emerged, focusing on attempts to remove the ‘brakes’ (Checkpoint Inhibitors) that the tumor places on the immune system, thus allowing T cells to enter the tumor and eliminate it. Researchers in this field were awarded the Nobel Prize in 2018 for this discovery.”
And does it work?
“In certain types of cancer. The method of removing the brakes, which opens the door for T cells to enter the tumor and eliminate it, has created a huge revolution. There have been notable successes, particularly with melanoma, where significant percentages of stage 4 cancer patients have been cured using this method. In 2016, I attended a lecture by Prof. James Allison, one of the two researchers who received the Nobel Prize for this discovery, and he showed a picture of a woman who came to him in 2002 with stage 4 cancer. Thanks to this method, she beat the cancer and was still alive at that time. It was incredibly moving.”
What do you see in the T cells that are aware of the tumor?
“It turns out that even when the immune system can’t deal with the tumor, the awareness of its existence changes the content of the T cells. For example, we see more T cells with a certain type of receptor. We don’t fully understand how it works yet, but we count the changes in the content of the T cells – changes that likely indicate this signal. Our research is in the field of information.”
Chat GPT joins the fight against cancer
Prof. Efroni’s lab recently succeeded in identifying such differences in a study on the T cells of ovarian cancer patients. “In a study that was recently published, we took blood samples from women with and without ovarian cancer, and we were able to distinguish between them based on the information we gathered from the T cells in their blood. The ability to diagnose cancer from a blood sample is extremely important because ovarian cancer is usually diagnosed late.”
What does your lab look like? Are there test tubes or computers?
“Some of the students do experimental work, but most of them work on machine learning. Once the blood samples arrive at the lab, they undergo a fairly long and complex process that ultimately turns them into tables. The students and I can then input the data and analyze it using machine learning. In practice, it looks like a massive table. In our recent research on ovarian cancer, we had about 100 blood samples from women, each containing 2 million T cells, so the table had 2 million rows. Each row represented a sequence, averaging 45 nucleotide bases, which are the building blocks of our DNA. That’s the material we analyze.”
How does machine learning come into play here?
“It’s a machine we created, and its job is sorting: the goal is to separate the two groups, healthy women and those with cancer. The field of machine learning has advanced greatly, and many students come to us to specialize in it.”
Does it help that this field has become so popular?
“Absolutely. In my post-doctorate more than 15 years ago, I had to manually write many of the functions that are now readily available because others have written them. Luckily, at Bar-Ilan, we have several top experts in the field, like Gal Chechik and Jacob Goldberg, among many others, who have been at the forefront of the field (Goldberg taught me during my PhD at Weizmann in 2002) and have made significant contributions. The progress in the field has really eased our work. For instance, in 2017, the tool known as ‘Transformer’ was introduced, which is the ‘T’ in Chat-GPT. The acronym GPT stands for Generative Pre-trained Transformer. This means that by 2020, we were already able to use this tool with relative ease.”
But the Transformer was developed for linguistic mediums, how does that fit in?
“The Transformer is based on the fact that the order of words in a sentence matters. What does Chat-GPT actually do? It tries to predict the next word. This allows us to use this tool to analyze sequences of amino acids that make up T cells, which essentially look like sequences of words and sentences. Just as the meaning of a sentence can change dramatically when you change the order of the words, the sequence in T cells is also crucial."
Please explain how it works.
“We have a very large collection of sequences from blood samples we’ve gathered, several hundred million such sequences. We then ask the Transformer, after it has learned these sequences: what will the next amino acid be? For us, amino acids are like words, and the Transformer predicts their order based on the sequences it already knows. Afterward, you show it the correct answer, and that’s how it learns: you hide the answer, ask it, and it responds, then corrects itself if necessary. This learning process is complex and lengthy because the sequences are very long, but it manages to analyze these sequences quite well.”
So the goal of the Transformer is to check if a sequence is unusual?
“Even though there’s potential to create as many sequences as there are grains of sand, a certain percentage of sequences are common to all humans. It’s not clear exactly what that percentage is; some researchers claim it’s 3%, while others argue it’s 20%. But the Transformer can analyze the sequence and determine whether it’s routine or rare.”
Huge Commercial Potential
There have been commercial companies that tried to extract information from blood samples in the past. How are you different from them?
“There’s an American company called Grail that tried to diagnose breast cancer using a blood sample. They raised funds in the past from people like Jeff Bezos and Bill Gates, but it didn’t work out so well for them. They were trying to find remnants of the tumor in the blood sample, but since we’re talking about just a few molecules, it’s very challenging. We’re not looking for tumor remnants; we’re looking for something that’s abundant in the blood – T cells.”
“There’s one company that’s very close to what we’re doing, and they are quite successful because during the COVID pandemic, they also developed diagnostics for the virus. They’re called ADPT, Adaptive Biotechnologies. Initially, they focused on T cell tumors, but they have since shifted to problems like viruses. This is a field with enormous commercial and scientific potential, though it’s still in its infancy.”
Why are you focusing specifically on ovarian cancer?
“We’re not focusing on a specific type of cancer; we’re dependent on the samples we can obtain. The reason we worked on ovarian cancer and breast cancer is that we were able to get blood samples for ovarian cancer. That’s also why we have studies on colon cancer, liver cancer, breast cancer, and COVID – because we were able to obtain blood samples.”
Is it really that difficult to obtain blood samples?
“The costs of collecting blood samples are enormous. For example, mammograms are done all the time – around one million mammograms a year are performed in Israel alone. In theory, it would seem simple to collect blood samples at the same time, but the cost of taking and storing blood samples at freezing temperatures is huge, around $1,000 per sample. Once, we managed to secure the funds and sent them to a company in the U.S. to collect 100 melanoma samples for us, but after a year, they returned the money because they couldn’t collect the samples. All of our research has been made possible thanks to our lab manager, Dr. Alona Zilberberg, who established connections with blood banks and clinicians and managed to collect over 1,000 blood samples.”
Prof. Efroni describes the difficulty in obtaining blood samples as the main obstacle for his research. “We have an ongoing problem obtaining blood samples. We have collaborations and grants aimed at this, but it’s still very challenging. When I arrived for my post-doctorate at the NCI, there weren’t enough cancerous samples, so the NCI collected 14,000 samples; those samples changed the face of research. Initially, the test data was stored on a single computer just beyond the wall of my office, but by the end of my post-doctorate, there were 400 people working in the building, all on the same project, thanks to those samples.”
What makes this research part of personalized medicine?
“Immunotherapy treatment based on inhibiting cellular entry checkpoints is part of personalized medicine. However, despite the enormous revolution in this field, the response rate is still relatively low, and some patients may experience severe reactions to the treatment. Our work aims to predict this in advance, so the treatment is given only to those who will benefit from it. So far, we’ve been successful in mice: we can predict which ones will respond well to the treatment and which will not.”
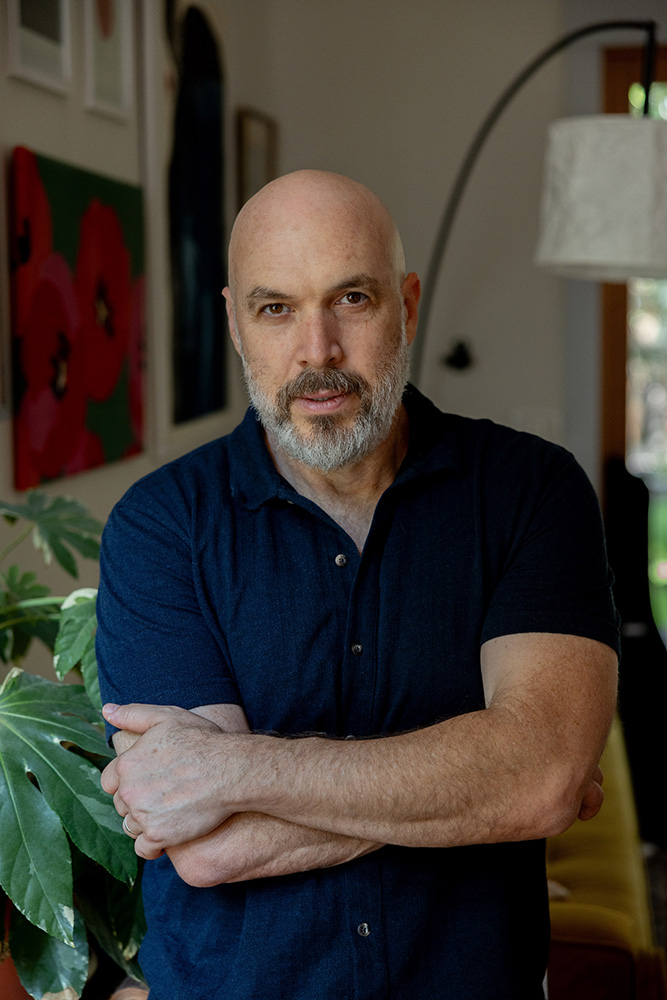
He left school at 15, and thanks to a lecturer from Bar-Ilan, discovered physics
Prof. Sol Efroni completed his bachelor’s degree in physics at Tel Aviv University, his master’s degree in cognitive sciences at the Hebrew University, and his PhD-focused on T cells-at the Weizmann Institute, in the field of immunology and computer science. He conducted his post-doctoral research at the National Cancer Institute (NCI) in Maryland, USA, and opened his lab at Bar-Ilan University in 2009.
Why did you choose to establish your lab at Bar-Ilan?
“After my post-doctorate, I wanted to return to Israel, and Bar-Ilan was incredibly kind in a way I hadn’t experienced at other universities where I studied and worked. But, in fact, there’s a deeper reason, which I only remembered recently. I left school at 15 – we were a group of eight close friends who were troublemakers, and they separated us into different classes. I refused to stay in school. At 16, I started studying physics at Bar-Ilan. Of course, I didn’t meet the admission requirements, but there was one professor, Prof. Moshe Gitterman, who opened the door for me. He spoke to the lecturers, asking them to let me into their classes and to grade my exams. I remembered Bar-Ilan fondly, and when they made me an offer, I accepted within just a few days.”
As a university that encourages collaboration, with which researchers do you collaborate?
“At Bar-Ilan, there are two other researchers working on repertoires, meaning on samples of T cells – Prof. Gur Yaari from bioengineering and Prof. Yoram Louzoun from the mathematics department. But in practice, most of my collaborations are with clinicians who can provide our lab with blood samples.”
Where do you see yourself in ten years?
“Hours pass slowly, but the years go by quickly. When I first arrived at Bar-Ilan in 2014, I was immersed in a different field, continuing the research from my post-doctorate. I didn’t take a moment to think if it was truly what I wanted to do. Only after I became a full professor did I stop and start exploring machine learning, a field that interests me more than anything else. So, I can’t predict what will interest me in ten years; what’s certain is that I would be very happy if we could show the results we’re achieving now on a much larger number of samples. I wish that for the entire field, not just for myself.”
Last Updated Date : 10/12/2024